Bayesian Inference on non-Gaussian Structural Vectorautoregressions
Published | Share
On the 15th of December, Jetro Anttonen will defend his doctoral dissertation “Bayesian Inference on non-Gaussian Structural Vector Autoregressions”.
In the dissertation, a complete framework for parametric Bayesian analysis of non-Gaussian structural vector autoregressions is developed. In particular, the developed framework allows for more general distributional assumptions than entertained in the previous Bayesian literature on structural vector autoregressions. Consequently, more information in the data can be exploited for statistical identification and the risk of model misspecification is diminished. The dissertation comprises an introductory chapter, providing some background and motivation, and three self-contained studies.
In the first study, a simple version of the framework is developed and discussed. The shocks are assumed homoskedastic and differential evolution Markov chain algorithm is proposed as a relatively simple, but robust and sufficiently efficient, solution to the estimation of non-Gaussian structural vector autoregressions. The asymmetry of the structural shocks is exploited for identification, seemingly for the first time in the Bayesian literature on non-Gaussian structural vector autoregressions. Extensive simulation studies underline the importance of this feature for the strength of identification. In an empirical application to U.S. monetary policy, the applicability of narrative sign restrictions to labeling the statistically identified shocks is showcased and the monetary policy is successfully identified. Results suggest there to be a strong negative response of real activity to a contractionary monetary policy after a few months’ delay, as expected.
In the second study, the framework is further developed, as different shock volatility structures are thoroughly discussed and their implementation illustrated. Novel identification results are provided and the so-called NUTS sampler combined with automatic differentiation is proposed as a general solution to the estimation of non-Gaussian structural vector autoregressions. Specifically, it is shown that although non-Gaussianity should be allowed for, Gaussianity needs not be ruled out for valid Bayesian inference and even mutual shock dependence can be allowed for, to a some extent, under relatively standard assumptions. Practical implementation of the proposed methods is thoroughly discussed and both simulation studies and an empirical application to U.S. fiscal policy provide evidence of the practical performance of the proposed framework.
Finally, in the third study of the dissertation, the developed framework is put to test in an extensive empirical application. A ten-variate model from the previous literature studying the relationship between credit growth and output in the U.S. is put under scrutiny. It is shown that some conclusions in the previous literature are misplaced, due to less robust estimation algorithms and the inability of the previously existing methods to exploit all the relevant information in the data. Particularly, as opposed to null results in the previous literature, business credit expansion shocks are found to have a significant negative effect on output that cannot be accounted to an endogenous monetary policy response.
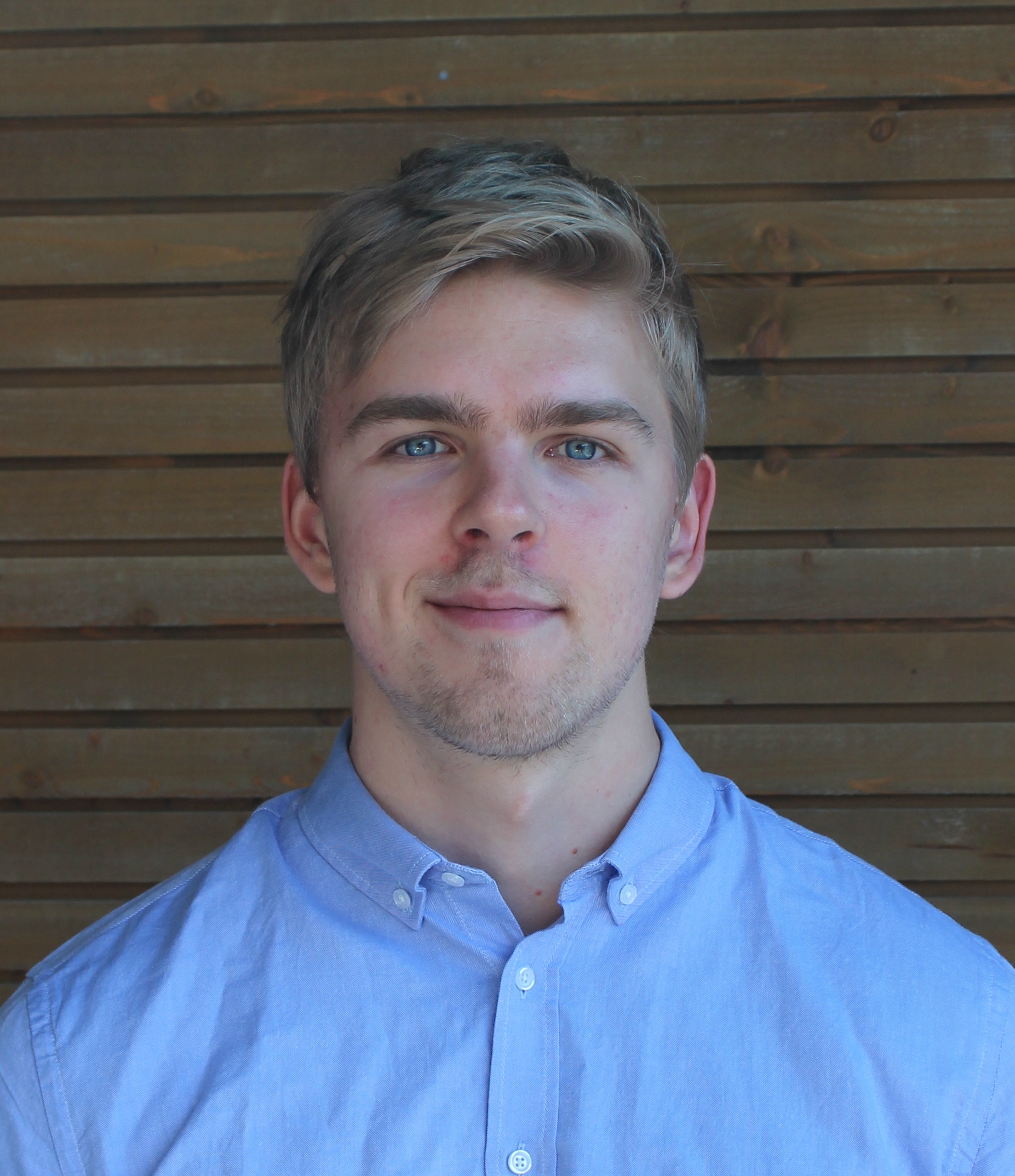
Contact Jetro Anttonen
Email: jetro.anttonen@helsinki.fi
X: @JetroAnttonen